USING MACHINE LEARNING ALGORITHMS IN TRAINING FUTURE COMPUTER SCIENCE TEACHERS
DOI:
https://doi.org/10.59787/2413-5488-2025-49-1-52-66Abstract
The beginning of the application of artificial intelligence in various areas of society in recent years has put forward the requirements for the introduction of innovative technological methods into education. Improving the knowledge of future computer science teachers is being connected with the directions of modern artificial intelligence. In the process of training computer science teachers in the higher education system of the country, new disciplines are taking a place in the composition of educational programs. In particular, the direction of artificial intelligence machine learning is included in educational programs for training computer science teachers and gives positive results. In the educational process, the theoretical foundations of machine learning are being practically implemented in modern programming languages; in particular, the Python language with the use of the ChatGPT chatbot and the R language for programming scientific problems are being used.
References
Acquah, B.Y.S., Arthur, F., Salifu, I., Quayson, E., & Nortey, S. A. (2024). Preservice teachers’ behavioural intention to use artificial intelligence in lesson planning: A dual-staged PLS-SEM-ANN approach. Computers and Education: Artificial Intelligence, 7, 100307. https://doi.org/10.1016/j.caeai.2024.100307
Baikulakov, Sh., & Belgibayev, Z. (2021). Analysis of consumer credit risks using machine learning algorithms. Available at: https://nationalbank.kz/file/download/69067
Ben Said, Mouna; Hadj Kasem, Yessine; Algarni, Abdulmohsen; Masmoudi, Atef. Early prediction of Student academic performance based on Machine Learning algorithms: A case study of bachelor’s degree students in KSA. Education and Information Technologies. Т. 29. V. 11. Pp. 13247 – 13270. 2024. https://doi.org/10.1007/s10639-023-12370-8. http://surl.li/dyyfgk.
Esekeshova, M. (2018). Fundamentals of Scientific and Pedagogical Research. Astana: Foliant.
Iskakov, R. O. (2015). Application of machine learning algorithm for document clustering. Semipalatinsk.73b.
Kudaibergen, K. (2023). Can artificial intelligence replace teachers? https://inbusiness.kz/kz/news/zhasandy-intellekt-mugalimdi-almastyra-ala-ma
Laakel, H., Lamarti, S. M., Achtoun, Y., & Tahiri, I. (2024). Comparative analysis of feature selection and extraction methods for student performance prediction across different machine learning models. Computers and Education: Artificial Intelligence, 7, 100301. https://doi.org/10.1016/j.caeai.2024.10030
Machine Learning in Education: Benefits and Opportunities. (2024, July 1). Intellias.: https://intellias.com/benefits-of-machine-learning-in-education/
Mukhamediyeva, K. B. (2020). Machine learning in improving the educational environment. Issues of Education and Social Development, 4(13), 70-77.
NCPC "Orleu". Features of using artificial intelligence tools in the learning process. Republican information and analytical journal "Modern Education". http://surl.li/fihsmu.
Okoye, K., Nganji, J.T., Escamilla, J., Hosseini, S. (2024). Machine learning model (RG-DMML) and ensemble algorithm for prediction of students’ retention and graduation in education. Computers and Education: Artificial Intelligence, 6, 100205. https://doi.org/10.1016/j.caeai.2024.100205http://surl.li/ykdykw
Serik, M., Nurbekova, G., Mukhambetova, M., & Zulpykhar, Z. (2022). The educational content and methods for big data courses including big data cluster analysis. World Transactions on Engineering and Technology Education, 20(3), 203-208 https://www.scopus.com/record/display.uri?eid=2-s2.0-85136273484&origin=resultslist
Serik, M., Nurgaliyeva, S., & Balgozhina, G. (2022). Introducing robotics with computer neural network technologies to increase the interest and inventiveness of students. World Transactions on Engineering and Technology Education, 20(1), 33-38. Retrieved from https://www.scopus.com/record/display.uri?eid=2-s2.0-85128865055&origin=resultslist
Tan Zh., Wang S., Yang Z., Chen G., Huang, X., Sun M., Liu Y. (2020). Neural machine translation: A review of methods, resources, and tools. AI Open, 1, 5-21. https://www.sciencedirect.com/science/article/pii/S2666651020300024
Tokzhigitova, N. K., Zhumadillaeva, A. K., & Alimbekova, N. A. (2022). Machine Learning Data Analysis. Pavlodar: Toraighyrov University.
Weegar, R., & Idestam-Almquist, P. (2024). Reducing workload in short answer grading using machine learning. International Journal of Artificial Intelligence in Education, 34, 247-273. https://doi.org/10.1007/s40593-022-00322-1
Will AI Replace Teachers? The Future of Education. (2024) https://www.e-learningpartners.com/blog/will-ai-replace-teachers-the-future-of-education
Xiao, S., Li, J., Lu, J., Huang, S., Zeng, B., Wang, S. (2024). Graph neural networks for multi-view learning: a taxonomic review. Artificial Intelligence Review, 57, 341. https://doi.org/ 10.1007/s10462-024-10990-1 https://clck.ru/3EhJsV
Yerlanova G., Serik M., Kopyltsov A. (2021) High performance computers: From parallel computing to quantum computers and biocomputers. Journal of Physics: Conference Series. No.1889(3). 32-36. https://doi.org/10.1088/1742-6596/1889/3/032032
Zhu W., Wang X., Xie P. (2022). Self-directed Machine Learning. AI Open, 3, 58-70. https://www.sciencedirect.com/search?qs=machine%20learning&pub=AI%20Open&cid=777606
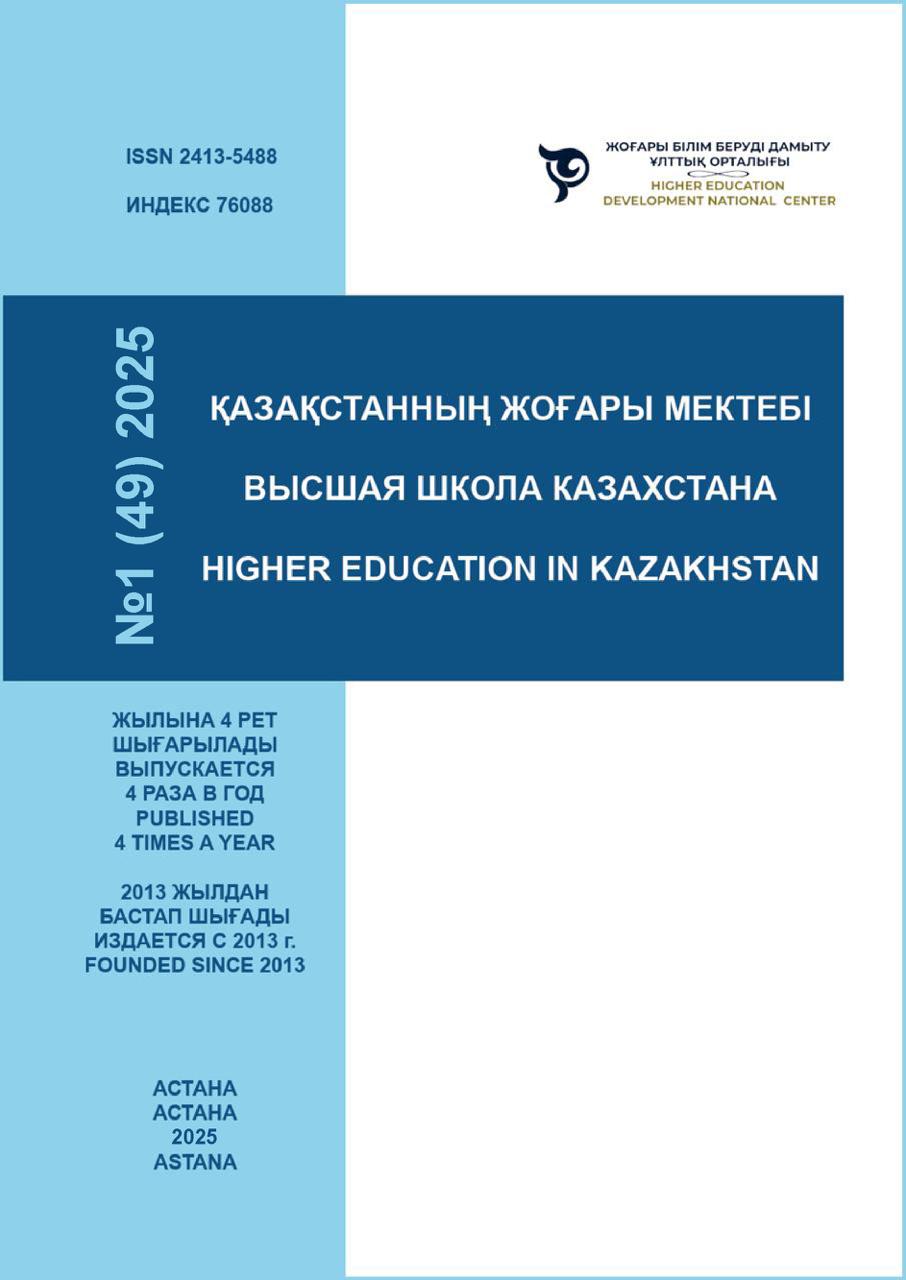
Downloads
Published
Issue
Section
License
Copyright (c) 2025 Scientific and analytical journal "Higher school of Kazakhstan"

This work is licensed under a Creative Commons Attribution-NonCommercial 4.0 International License.